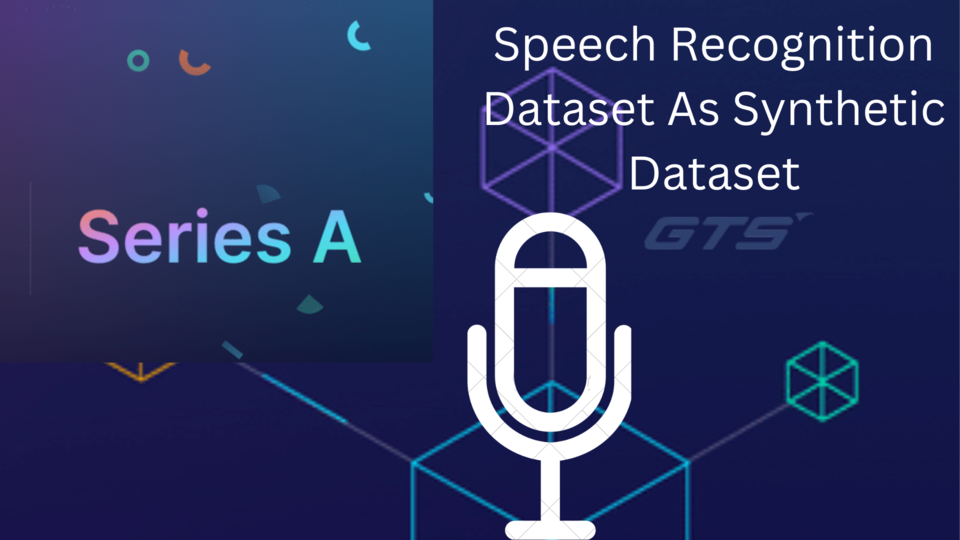
The rapid expansion of vocal technologycan be attributed to a number of reasons. One of them is the rise in the use technology, such as the advancement of biometrics that are operated by voice as well as voice-driven navigation systems as well as the advancements with the development of machine-learningmodels. Let's explore the latest technology and get to know its functions and applications.
As technology advances it has led to a difficulties in obtaining the necessary AI Training Datasets for ML models. To make up for this, a lot of synthetic or artificial data is created or simulated to help train models using ML. Primary data collection, though extremely reliable, can be expensive and time-consuming, and therefore there is a rising demand for simulated data that might or not be reliable and mimic actual experiences. This article merely attempts to look at the advantages and disadvantages.
In just a little over twenty years, the voice recognition technology has exploded in popularity. What does the future have in store? In the year 2020, the global technology for voice recognition was $10.7 billion. It is expected to explode into $27.16 billion in 2026. This will grow at an average CAGR of 16.8 percent from 2021 to 2026.
What is Voice Recognition?
The term "voice recognition," also known by its name speaker recognition, is program that has been developed to recognize the voice of a person, decode it, as well as authenticate the voice an individual by their unique voiceprint.
The program assesses the biometrics of a person's voice by scanning their voice and comparing it with the necessary speech command. It analyzes with care the frequency and pitch, accent, intonation, and the stress that the person speaking.
What's the benefit of synthetic data? And when should you use it?
The data is created by algorithms instead of being generated through real-world events. The real data is observed in the real world. It can be used to gain the most valuable insight. Although real data can be valuable however, it can be costly and time-consuming to collect and difficult to access because of privacy concerns. Synthetic data hence becomes a secondary/alternative to real data and can be used to develop accurate and advanced AI models. The artificially created data is combined with real data to create an enhanced data set that does not suffer from the flaws inherent to real data.
Synthetic data can be used to test a new system in which real data is either unavailable or is biased. Synthetic data may also be used to supplement real data, which is limited and unshareable, inaccessible, and inaccessible.
How Does Voice Recognition Work?
The technology for speech recognition goes through a series of steps before it is able to reliably identify the speaker.
It starts by converting the analog recordings into digital ones. To understand the question you're asking the voice assistant the microphone inside your device, will pick up your voice, transforms them into electrical currents, then converts these analog sounds into binary digital format.
As electrical signals are transferred through the Analog-toDigital Converter the program begins to collect signals of voltage fluctuations within certain areas in the electrical current. The samples are tiny in length - they are just a few thousandths of a second. The voltage will determine whether the converter assigns binary numbers in the input data for Audio Transcription.
In order to decipher the signals the computer program requires an elaborate database of digital vocabulary, syllables and phrases or wordsand an efficient method of comparing the signals with information. The audio-to-digital converter is able to compare the sounds of the database to the digital audio converter by with a pattern recognition function.
Why Use Synthetic Data?
The acquisition of large quantities of high-quality data to build models within the set time frame is a challenge for many companies. In addition the manual labeling of data can be a lengthy and costly process. This is why creating synthetic data can assist businesses overcome these obstacles and build reliable models in a short time.
Synthetic data lessens dependance on the original information and reduces the necessity to collect it. It is a more simple to produce, more cost-effective and efficient method to create data sets. A large amount of quality data can be produced within a shorter period of time than real-world data. It is particularly useful to create data based on edge events , which are rare events. Furthermore the synthetic data can be labeled, and then annotated as it is generated which reduces the time needed to label the data.
When privacy or data safety are the primary security concerns, synthetic datasets can be utilized to reduce the risk. Real-world data must be made anonymous to qualify to use it as for training purposes. Even with an anonymization process that removes identifiers from the data however, it's still possible for a variable to serve as an identifier variable. It is not the scenario with data that is synthetic since it is not inspired by a real person or an actual incident.
The process of identifying and authenticating the identity of a person by analyzing the voice of a person. The process is based by assuming that no two people can sound exactly the same due to the different larynx sizes, their form that their vocal tracts take and many other factors.
The accuracy and reliability of the speech or voice recognition software depend on the training method tests, databases, and training utilized. If you've got an innovative idea to develop software for voice recognition contact GTS to discuss your database and training requirements.
You can get an authentic top-quality, secure, and safe Audio Datasets that you can use to test or train the machine-learning or natural model for processing of languages.